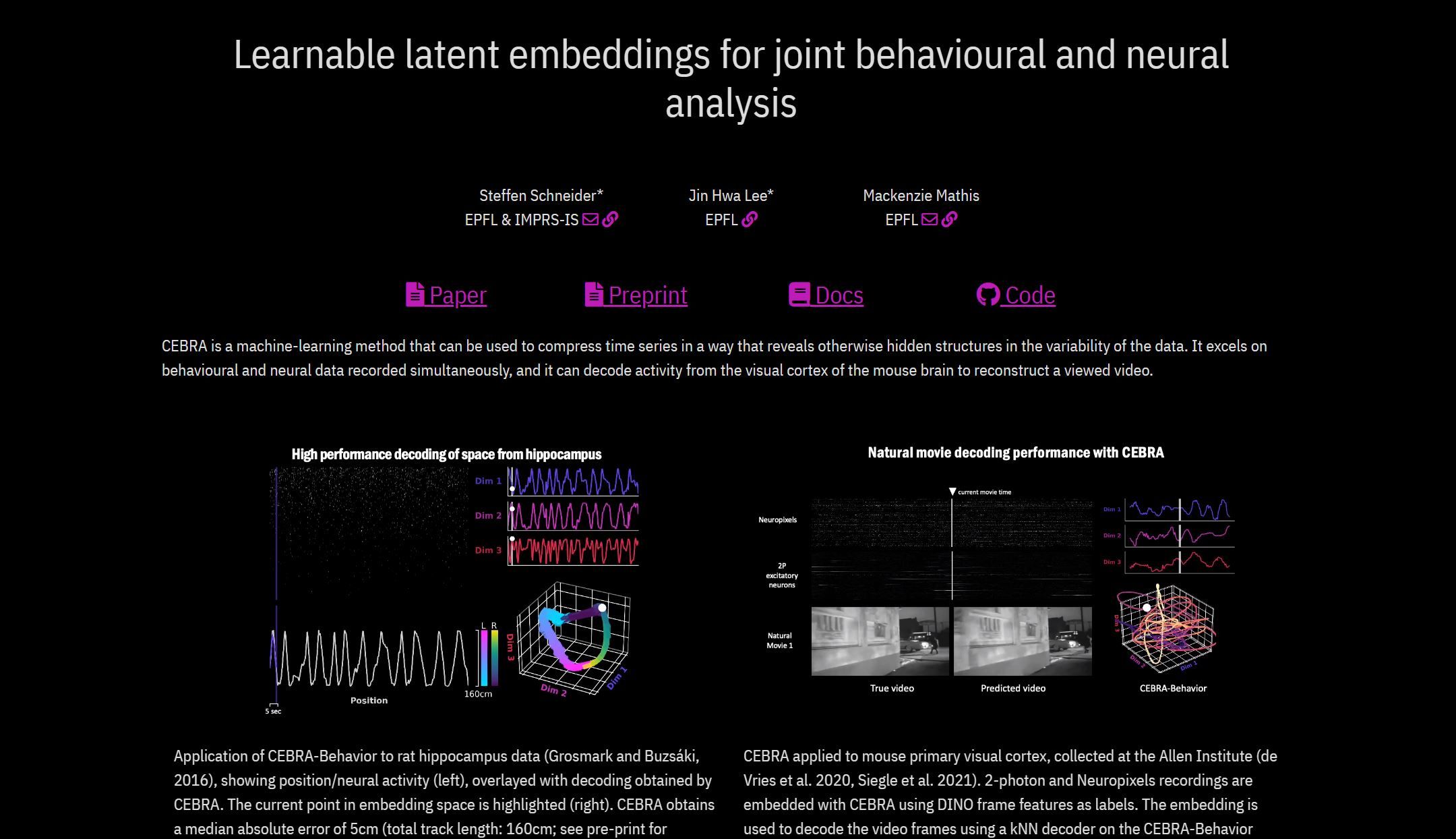
Cebra
Cebra: Learnable Latent Embeddings for Joint Behavioral and Neural Analysis
Cebra is a machine learning tool that utilizes non-linear techniques to create consistent and high-performance latent spaces from joint behavioral and neural data recorded simultaneously. It offers several key features:
- 🧠 Neural Latent Embeddings: Enables hypothesis testing and discovery-driven analysis.
- 🎯 Validated Accuracy: Proven efficacy on calcium and electrophysiology datasets, sensory and motor tasks, and simple or complex behaviors across species.
- 🔀 Multi-session and Label-free: Can be used with single or multi-session datasets and without labels.
- 📊 High-accuracy Decoding: Provides rapid decoding of natural movies from the visual cortex.
- 💻 Code Availability: Access the tool’s code on GitHub and read the pre-print on arxiv.org.
Use Cases
- 🔬 Analyze and Decode Behavioral and Neural Data: Uncover underlying neural representations through the analysis and decoding of behavioral and neural data.
- 🧠 Map and Uncover Complex Kinematic Features: Explore and understand complex kinematic features in neuroscience research.
- 🔁 Produce Consistent Latent Spaces: Generate consistent latent spaces across various data types and experiments.
Conclusion
Cebra is a valuable tool for neuroscientists seeking to analyze and decode behavioral and neural data. By leveraging non-linear techniques, it enables the exploration of underlying neural representations involved in adaptive behaviors. With its validated accuracy, multi-session and label-free capabilities, high-accuracy decoding, and code availability, Cebra empowers researchers to gain deeper insights into the complex workings of the brain.
FAQ
Q: Can Cebra be used with different species?
A: Yes, Cebra has been proven effective across species in various behavioral and neural tasks.
Q: Is labeling required for using Cebra?
A: No, Cebra can be used with both labeled and label-free datasets.
Q: Where can I access the code for Cebra?
A: The code for Cebra is available on GitHub, and you can also find the pre-print on arxiv.org.
See more Research AI tools: https://airepohub.com/category/research